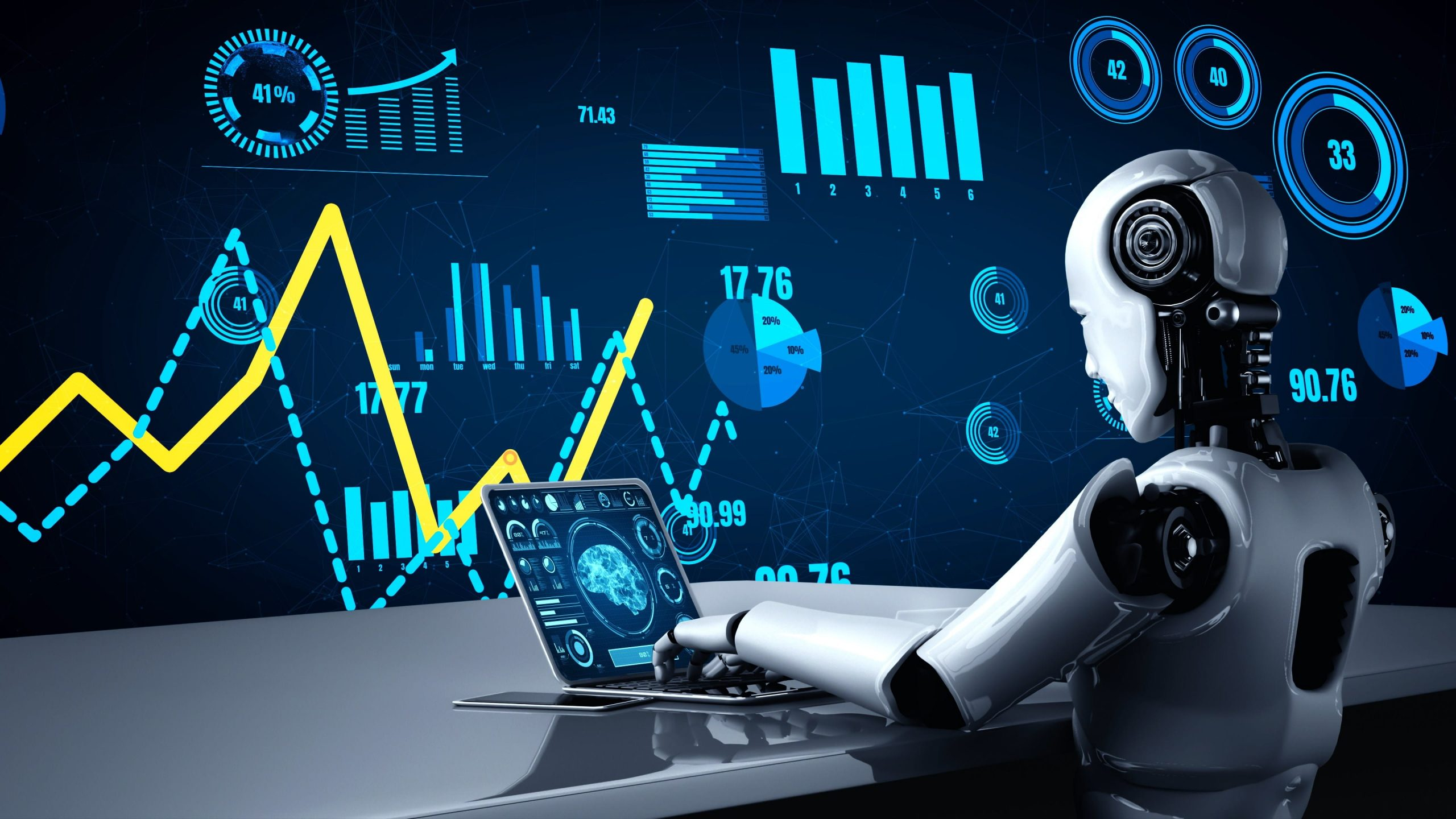
AI trading – Finance meets technology’s core
AI is increasingly influencing different areas of finance, including credit assessment, fraud prevention, portfolio handling, and risk evaluation. Yet, its most significant impact is seen in trading. AI’s capacity to handle and analyze large data sets, recognize patterns, and execute rapid decisions is revolutionizing the dynamic financial market landscape.
Traditionally, trading has been the domain of human analysts and traders who rely on their experience, intuition, and mental models to make investment decisions. However, the sheer volume and velocity of financial data in today’s markets have made it increasingly challenging for humans to keep pace. This is where AI comes in, with its ability to crunch numbers, spot opportunities, and execute trades at a speed and scale beyond human capacity.
Core of AI trading
- Machine learning – Machine learning algorithms automatically learn patterns and relationships from historical market data and use this knowledge to make predictions and inform trading decisions. Techniques such as regression, decision trees, and neural networks are commonly used in AI trading models.
- Natural language processing (NLP) – NLP allows AI systems to process and interpret human language data, such as news articles, social media posts, and earnings call transcripts. By analyzing this unstructured data, AI gains valuable insights into market sentiment, company fundamentals, and geopolitical events that can impact asset prices.
- Big data analytics – AI trading systems rely on the ability to process and derive insights from massive datasets, often in real-time. This requires robust data infrastructure, including distributed storage systems, streaming analytics engines, and high-performance computing clusters.
- Reinforcement learning – Reinforcement learning is a branch of AI where agents learn to make optimal decisions through trial-and-error interactions with an environment. In trading, reinforcement learning algorithms learn to navigate market conditions and optimize portfolio allocations based on rewards. For quantum ai canada check quantumai.bot.
By leveraging these core technologies, AI trading systems can uncover hidden patterns, predict future price movements, and adapt to changing market regimes. They can execute complex, multi-asset strategies quickly and continuously learn and improve. While AI has the potential to revolutionize trading, it also presents significant challenges and risks. The primary concern is the potential for AI systems to amplify market volatility and contribute to flash crashes, as seen in past incidents such as the 2010 Flash Crash. AI models’ opaque and complex nature also raises questions about transparency, accountability, and fairness.
The widespread adoption of AI in trading could lead to an arms race dynamic, where firms compete to develop ever-more sophisticated algorithms, leading to a winner-take-all market. This could concentrate risk and create new sources of systemic fragility. There is a growing need for research into explainable AI, risk management frameworks, and regulatory oversight of AI in financial markets to address these challenges. Collaborations between academia, industry, and policymakers will be crucial in developing best practices and standards for the responsible use of AI in trading.
The intersection of finance and technology is giving rise to a new era of AI-driven trading, where machines are becoming increasingly sophisticated in navigating complex markets. As the core technologies behind AI trading continue advancing, we expect to see more widespread adoption and innovation in this space. The rise of AI in trading also presents significant challenges that must be carefully managed. Developing robust governance frameworks and ethical standards will ensure that AI is used responsibly and in the best interests of all market participants.